The HETT Unexpected Innovation Awards returns in November 2022 to celebrate the very best in experimental design, collaboration, and leadership in digital healthcare.
The event showcases champions of digital health innovation and highlights some great stories of transformation. The awards bring together the health and care ecosystem and highlights best practice examples to inspire more to do the same.
The Data Innovation Award recognises digital products or services that demonstrate measurable impacts and outcomes delivered in big data projects over the last year.
The four shortlisted submissions for this category are:
CogStack Team
Hospital Electronic Health Record (EHR) systems hold rich patient data, but they are messy, incomplete, and often difficult to access. It is estimated that 80% of the data is unstructured, and often free-form text written by care providers in admission notes, care progress notes, discharge summaries, radiology and pathology reports, and other intervention notes.
Most hospitals hold this data across disparate, disconnected databases, each with their own schema, connectivity method and dataset idiosyncrasies. Gathering and filtering the required data for an audit or report can involve laborious, error-prone, manual reading of clinical notes in Word docs, PDFs and hand-written notes.
This leaves much data underutilised, leading to more expensive, inefficient processes, the returns on investment for the digitisation of records unrealised, and ultimately overall worse patient care.
An interdisciplinary team from King’s College London, King’s College Hospital, University College London, University College Hospitals and the South London and Maudsley NIHR Biomedical Research Centres have developed a fundamental infrastructure, CogStack, that supports real time ingestion, harmonisation and enterprise level searching, alerting, visualisations, and dashboards across an entire hospital's EHR.
CogStack is an ecosystem of loosely coupled, open-source, NHS home-grown technologies that is integrated and deployed at five NHS trusts with ten more trusts in queue. It has ingested 12 million documents spanning 20 years across multiple databases. The natural language processing technology structures the free-text portion of the record for any clinical terminology.
Major funding has been awarded by several agencies, and the platform has been recognised in government reports to the Chief Medical Officer and keynote speeches by the Health Secretary.
WNB AI Tool - Alder Hey Children's NHS Foundation Trust
Non-attendance for appointments often exacerbates health inequalities for children and young people. Over the period 2018-2019 in England, more than 15% of the missed appointments were for patients up to 19 years old.
Children who do not attend are referred to as ‘was-not-brought’ or WNB. WNBs are likely to be generated from children and young people in a vulnerable classification where there is a need to improve health outcomes and reduce wellbeing risk factors. Encouraging early attendance is critical as child mortality can be largely avoided through timely healthcare interventions.
The Alder Hey Innovation Team has developed an artificial intelligence tool, which includes health inequality variables, to identify individuals from the most vulnerable backgrounds, who are at risk of not attending their agreed appointments.
The WNB AI tool provides paediatric services with cutting-edge technology that automatically identifies risk factors which a human clinician would need to amass from multiple sources.
Once a patient has been identified as likely to not attend, there can be additional intervention with the patient to understand the barriers to access.
The AI algorithm provides an individualised risk score for each patient, which enables each specialism to adapt its approach. Clinicians can use the score to stratify their approach according to clinical risk, which ensures resources are used effectively.
The predictive model developed has an accuracy (AUROC) of 80%, and following successful deployment, the National Paediatric Accelerator invested £1m to deploy the tool to a further ten NHS hospital locations.
RPA EDYS Team - Alder Hey Children's NHS Foundation Trust
Since April 2021, Alder Hey has implemented nine RPA (Robotic Process Automation) processes with significant efficiency savings, improved data quality of patient and staff records, as well as a reduction in delays in accessing services.
The team was asked to use RPA to log first appointments for the EDYS (Eating Disorders) team. Whilst the service is relatively small, it was oversubscribed, and an inefficient administration process existed. Phone calls would bounce between the service and families. It is never ideal when people receive phone calls during working hours from ‘No Caller ID’.
It is vital that the appointment slots are booked and the patient attends to ensure they are seen at the right time. The team set up an automated process which has saved 13 minutes of administrative time per referral. It also created a more positive patient and family experience when it comes to accessing these urgent appointments.
This appointment booking process enables families to accept or reject an appointment via text message with confidence that the appointment will be booked almost instantly, preventing families having to phone multiple times to book an appointment.
The improved staff experience facilitates better workflow and gives back time to spend on more complex tasks, removing the more repetitive and time-consuming manual tasks. The scope for scaling this process is potentially limitless, releasing time back to teams and providing a more streamlined service to young people and families.
Long Stay Risk Score - Gloucestershire Hospitals Trust
A persistent problem facing hospitals is when medically fit patients cannot be discharged because of a lack of available care at home or in the community.
Evidence shows that those who stay for more than 21 days in hospital have a 20% mortality rate during or soon after their hospital stay (compared to 3% of shorter admissions). For example, 27% of long stay patients became unwell again compared to 11% overall. 10 days of bed rest in hospital leads to the equivalent of 10 years of ageing in a patient over 80 years. When those patients finally leave hospital, they often need even more care because of the deterioration they’ve experienced.
What if these patients could be identified before admission? What if clinicians could be alerted to their risk of long stay the minute they arrived in hospital?
The Gloucestershire Hospitals Trust decided to develop a Long Stay Risk Score using machine learning that can be viewed on the EPR as soon as a new patient arrives at the hospital.
Working with NHSx and Polygeist, they developed an algorithm to predict the risk of a long stay in hospital. The team utilised more than 1 million anonymised patients records, with data spanning seven years, to develop the tool.
The project has been a success, demonstrating good prediction of patient length of stay and excellent risk stratification based on this and other factors. It will lead to a multitude of benefits and can be rolled out across any NHS trust.
Visit the HETT Unexpected Innovation Awards 2022 website for more information about the categories and the upcoming awards ceremony.
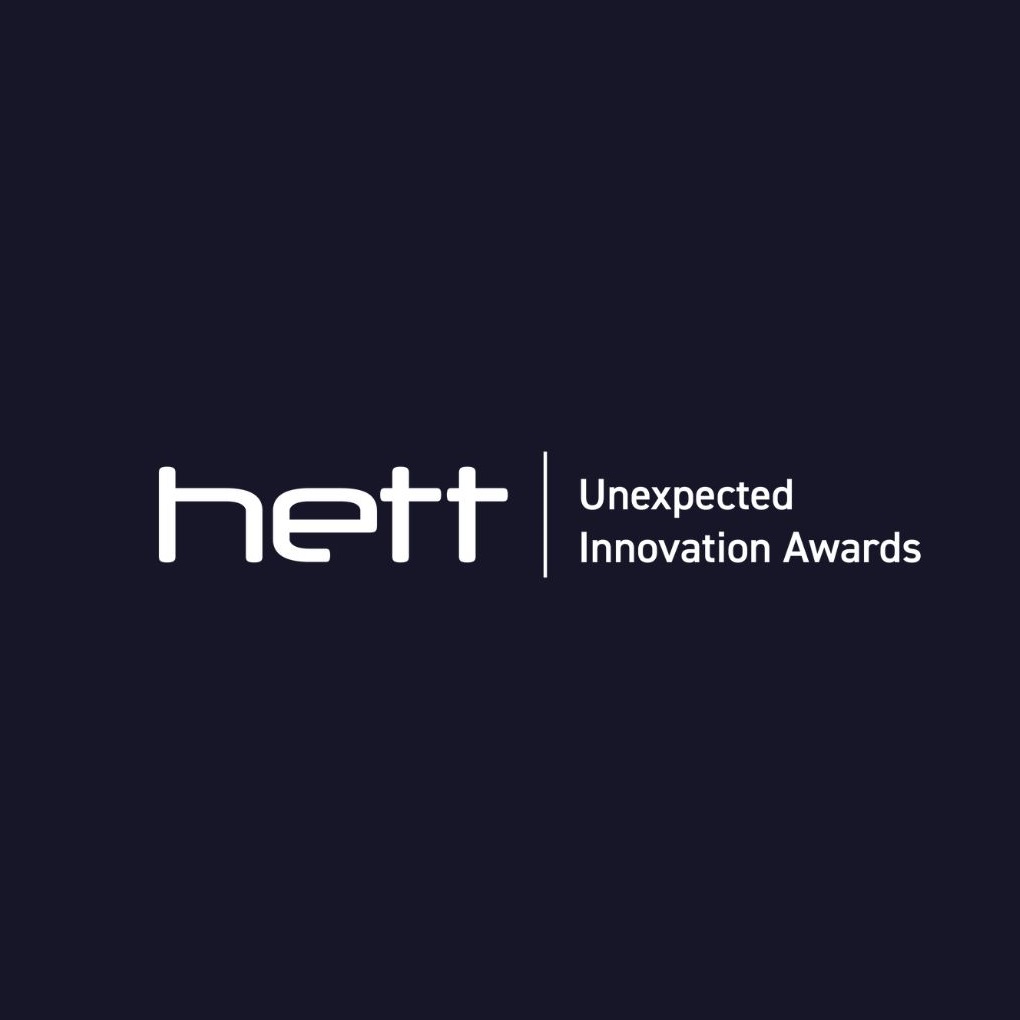
The HETT Unexpected Innovation Awards will take place on 23 November 2022 to celebrate the very best in experimental design, collaboration, and leadership in digital healthcare.